The eTRANSAFE consortium is happy to invite the world-wide scientific community to the Open Innovation Modelling Challenge. Consortium partners, Universitat Pompeu Fabra and University of Vienna, developed model building tools which allow easy transfer and validation of computational models at other partner’s premises, including EFPIA companies. The focus of this challenge lies on off-target modelling.
The best model(s)/results will be published and promoted by the eTRANSAFE consortium.
Registration is closed.
Start of Challenge: 30th of November 2021
End of Challenge: 28th of February 2022
Decision & Announcement of Winner: 29th of April 2022
The models shall be built only with one of these eTRANSAFE tools:
Two workshops were held on 17th of November to learn more about these tools. You may listen to the recordings of these sessions at the following links:
General Guidelines:
- You will be provided with sample data sets upon registration to the challenge. You are allowed to add/modify these data sets with your own data provided that you comply with the provisions of the below guideline number 6.
- Use only the abovementioned eTRANSAFE tools for the model building – UNIVIE Sandbox/KNIME Workflow & Flame.
- Submit max. two classification models*
- Use the pre-defined IDG-family threshold**
- Only submit classification models of the following six Off-targets:
- Adenosine A1 receptor (CHEMBL226, P30542)
- Dopamine D1 receptor (CHEMBL2056, P21728)
- Serotonin 1a (5-HT1a) receptor (CHEMBL214, P08908)
- Serotonin 2b (5-HT2b) receptor (CHEMBL1833, P41595)
- Cyclooxygenase-2 (CHEMBL230, P35354)
- Androgen Receptor (CHEMBL3072, P15207)
- Allowed additional Data Sources: Public databases as well as in-house data (as long as the data are not proprietary) ***
- Only non-commercial descriptors can be used
- Only classifiers, which are available from scikit learn can be used (only applies for Sandbox)
- Submit the model as well as the used training set
- Best model is selected based on its performance towards organizers´ in-house data ****
- The name of the winner will be made publicly available on the eTRANSAFE website.
- Subject to further discussions with the winning groups, the best performing models will be made available on the eTRANSAFE project portal (ToxHub).
*It is possible to submit two classification models in total. You are free to provide two classification models for the same target as well as for two different targets.
** Please, use the pre-defined threshold according to the IDG-family. You can find the values in the target list.
*** Please make sure that the used data are not proprietary, since the data of the best performing model will be published. Also, when using public databases, please make sure that the usage of these data is in agreement with the database license.
**** The performance will be decided based on the dataset provided by the consortium. The first criteria for the model performance is the correct prediction of positives of the test compounds, assessed by the precision and the recall. In a second evaluation step, the specificity as well as the balanced accuracy will be judged.
eTRANSAFE Tools
UNIVIE Sandbox / KNIME Workflow
The UNIVIE Conformal Prediction Modelling Toolbox is used to generate conformal prediction models. The tool includes a customized feature selection as well as a hyperparameter search. Finally, the user receives an optimized model based on the best-balanced accuracy. The performance of the model can be determined on statistical metrics and visualized with a confusion matrix. Further, the models should be validated by using consortium data for testing.
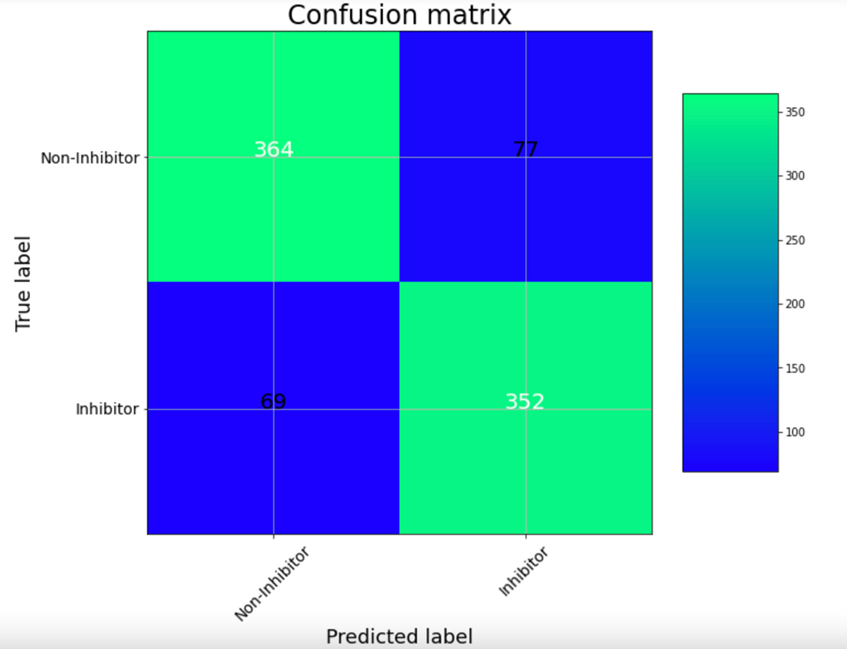
Flame
Use of Flame for the development, management, and application of predictive models.
Flame is an open-source framework for the development of predictive models. QSAR models can be generated starting from a collection of compounds annotated with biological activities. Flame implements customizable workflows that can be used to standardize the chemical structures, generate molecular descriptors, build machine learning models and validate them. Once generated, the models can be used as “predictive engines” that can be easily interchanged or deployed to predictions servers.
In this workshop, you will learn how to build QSAR models in Flame, using diverse molecular descriptors and machine learning algorithms. You will also manage these models, documenting and exporting them. The models generated by you will be tested by predicting the properties of series of test compounds and analysing the results.
More advanced model-building techniques, like ensemble models and advanced model customization, will also be introduced in this session.
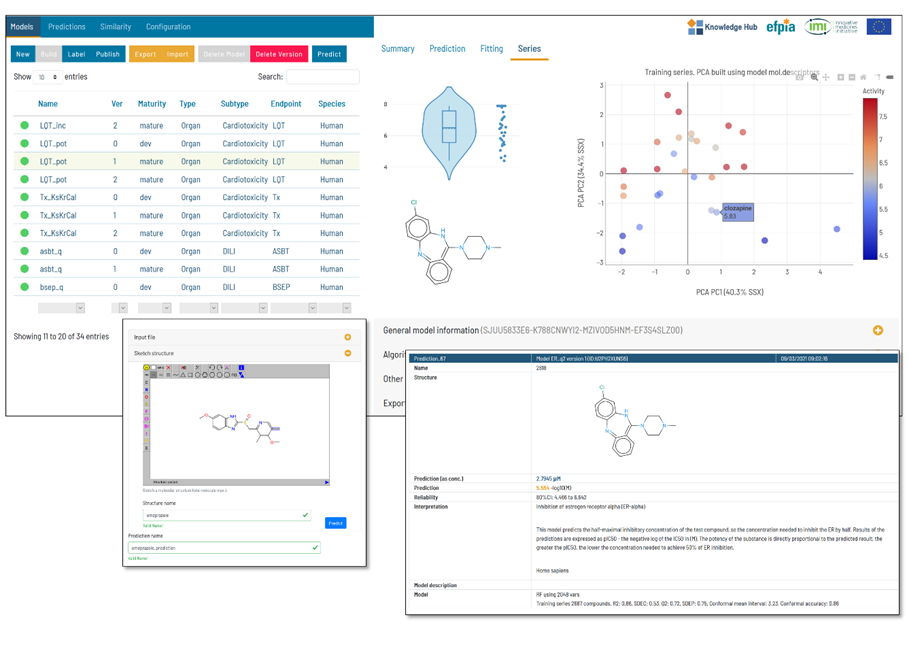